The question “do you need a data scientist?” came up a lot when I was a management consultant for a global firm that successfully incubated data science within a few enterprise organizations. It’s hard. The discussion is hard and the culture clash for data scientists is hard. Many approach data science as some dark magic from Hogwarts. It’s not. Investigating a hypothesis takes time. Spontaneously generating data and building a model against that data doesn’t work. Understanding who you need and how they will fit into your organization is challenging. Where do we put them? Who do they interact with? What is the hand-off? Who do we structure around the project? How do you execute a project? Even better, how do we make MONEY? Yet, before we go there, perhaps we should step back a bit and think of this as a strategic question. Because maybe you do need a data scientist and maybe you don’t.
If you are thinking about whether or not you need a data scientist, then here are some questions and insights to consider.
How accessible is your data?
- Algorithms are not the problem. Understanding what data goes into those algorithms is the crux of the issue. This requires accessible data.
- There are many access patterns in data science. These patterns include discovery, development, deployment, and maintenance. Getting to an infrastructure and data lifecycle that supports these patterns takes time.
- Data Scientists ask a lot of questions about data. Asking questions on raw data is hard and time intensive. It is expensive to pay a data scientist to ask questions on raw data when you are doing an insights driven project. It is probably best to enhance your calm and bring them on board when your data is ready for witchcraft and wizardry.
- Focus on getting accessible quality data and solid reporting. Then worry about data science. You’ll save money and efficiency.
How vs. Why?
- If you start with data science and ask how you do it rather than why you need it, you end up solving a problem for the wrong use-case. For example, you may end up focusing on scale and then find out what you needed was effective sampling techniques.
- If you solve for why, how becomes easy.
Product or Project?
- Are you making a product or doing a 6 month project?
- Is the project being reused?
- A product that has a point of failure on a data pipeline is different from a project that needs the output of a data pipeline.
- A data scientist can certainly do a project and get insights, building an infrastructure that empowers a group of data scientists to drive insights takes a product mindset. Data reusability and accessibility are key.
- Data Scientists are product people. You can sell a product for a long time. It is hard to justify ROI on a data scientist for a short-term project that isn’t likely to be reused.
I firmly believe that everyone in the enterprise needs or will need data science at some point. Yet, finding a relevant product that requires data science is the hard part. Statistics and predictive modeling are not new. Throw in ad-hoc innovative culture, scale, and reusable data pipelines all feeding some user application and you might have data science. Maybe the question isn’t “do you need a data scientist?” but rather, “are you doing something right now that warrants data science?”
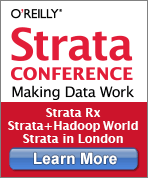
Strata Rx Health Data Conference: September 25-27 | Boston, MA
Strata + Hadoop World: October 28-30 | New York, NY
Strata in London: November 15-17 | London, England