- Flux: New Approach to System Intuition (LinkedIn) — In general, we assume that if anything is best represented numerically, then we don’t need to visualize it. If the best representation is a numerical one, then a visualization could only obscure a quantifiable piece of information that can be measured, compared, and acted upon. Anything that we can wrap in alerts or some threshold boundary should kick off some automated process. No point in ruining a perfectly good system by introducing a human into the mix. Instead of numerical information, we want a tool that surfaces relevant information to a human, for situations that would be too onerous to create a heuristic. These situations require an intuition that we can’t codify.
- Jumping to the End: Practical Design Fiction (Vimeo) — “Magic is a power relationship” — Matt Jones on the flipside of hiding complex behaviours from users and making stuff “work like magic.” (via Richard Pope)
- Predicting Daily Activities from Egocentric Images Using Deep Learning — aka “people wear cameras and we can figure out what they’re going to do next.”
- 402: Payment Required (David Humphrey) — The ad blocking discussion highlights our total lack of imagination, where a browser’s role is reduced to “render” or “don’t render.” There are a whole world of options in between that we should be exploring.
"Big Data" entries

Resolving transactional access and analytic performance trade-offs
The O’Reilly Data Show podcast: Todd Lipcon on hybrid and specialized tools in distributed systems.
Subscribe to the O’Reilly Data Show Podcast to explore the opportunities and techniques driving big data and data science.
In recent months, I’ve been hearing about hybrid systems designed to handle different data management needs. At Strata + Hadoop World NYC last week, Cloudera’s Todd Lipcon unveiled an open source storage layer — Kudu — that’s good at both table scans (analytics) and random access (updates and inserts).
While specialized systems will continue to serve companies, there will be situations where the complexity of maintaining multiple systems — to eke out extra performance — will be harder to justify.
During the latest episode of the O’Reilly Data Show Podcast, I sat down with Lipcon to discuss his new project a few weeks before it was released. Here are a few snippets from our conversation:
HDFS and Hbase
[Hadoop is] more like a file store. It allows you to upload files onto an arbitrarily sized cluster with 20-plus petabytes, in single clusters. The thing is, you can upload the files but you can’t edit them in place. To make any change, you have to basically put in a new file. What HBase does in distinction is that it has more of a tabular data model, where you can update and insert individual row-by- row data, and then randomly access that data [in] milliseconds. The distinction here is that HDFS is pretty good for large scans where you’re putting in a large data set, maybe doing a full parse over the data set to train a machine learning model or compute an aggregate. If any of that data changes on a frequent basis or if you want to stream the data in or randomly access individual customer records, you’re kind of out of luck on HDFS. Read more…
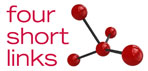

Four short links: 6 October 2015
System Intuition, Magic is Power, Predicting Behaviour, Payment Required

Specialized and hybrid data management and processing engines
A new crop of interesting solutions for the complexity of operating multiple systems in a distributed computing setting.
The 2004 holiday shopping season marked the start of Amazon’s investigation into alternative database technologies that led to the creation of DynamoDB — a key-value storage system that went onto inspire several NoSQL projects.
A new group of startups began shifting away from the general-purpose systems favored by companies just a few years earlier. In recent years, we’ve seen a diverse set of DBMS technologies that specialize in handling particular workloads and data models such as OLTP, OLAP, search, RDF, XML, scientific applications, etc. The success and popularity of such systems reinforced the belief that in order to scale and “go fast,” specialized systems are preferable.
In distributed computing, the complexity of maintaining and operating multiple specialized systems has recently led to systems that bridge multiple workloads and data models. Aside from multi-model databases, there are an emerging number of storage and compute engines adept at handling different workloads and problems. At this week’s Strata + Hadoop World conference in NYC, I had a chance to interact with the creators of some of these new solutions.
OLTP (transactions) and OLAP (analytics)
One of the key announcements at Strata + Hadoop World this week was Project Kudu — an open source storage engine that’s good at both table scans (analytics) and random access (updates and inserts). Its creators are quick to point out that they aren’t out to beat specialized OLTP and OLAP systems. Rather, they’re shooting to build a system that’s “70-80% of the way there on both axes.” The project is very young and lacks enterprise features, but judging from the reaction at the conference, it’s something the big data community will be watching. Leading technology research firms have created a category for systems with related capabilities: HTAP (Gartner) and Trans-analytics (Forrester).

Accelerating real-time analytics with Spark
Integration of the data supply chain is key to a reliable and fast big data analytics deployment.
Watch our free webcast “Accelerating Advanced Analytics with Spark” to learn about the architecture, applications, and best practices of Apache Spark.
Apache Hadoop is a mature development framework, which coupled with its large ecosystem, and support and contributions from key players such as Cloudera, Hortonworks, and Yahoo, provides organizations with many tools to manage data of varying sizes.
In the past, Hadoop’s batch-oriented nature using MapReduce was sufficient to meet the processing needs of many organizations. However, increasing demands for faster processing of data have emerged. These demands have been driven by recent developments in streaming technologies, the Internet of Things (IoT) and real-time analytics, to name just a few. These new demands have required new processing models. One significant new technology today that is being used to meet these demands and is gaining considerable interest and widespread support is Apache Spark. Spark’s speed and versatility make it a key part of today’s big-data processing stack in industries from energy to finance. Read more…
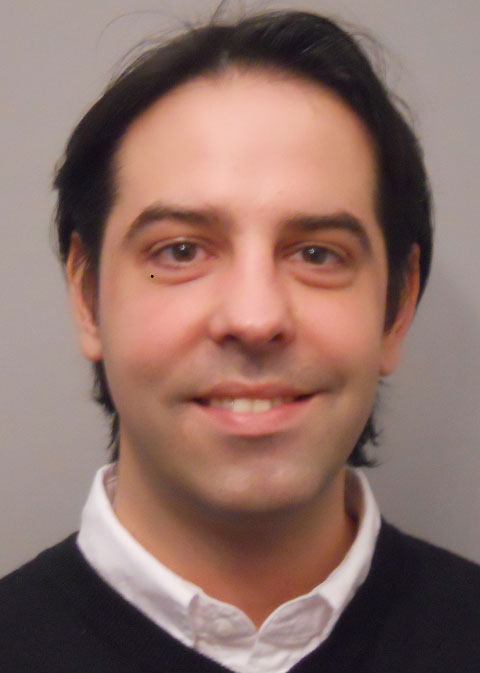
Translating data into knowledge
Best practices for data preparation — what you need to know before data analysis can begin.
Download “Data Preparation in the Big Data Era,” a new free report to help you manage the challenges of data cleaning and preparation.
Data is growing at an exponential rate worldwide, with huge business opportunities and challenges for every industry. In 2016, global Internet traffic will reach 90 exabytes per month, according to a recent Cisco report. The ability to manage and analyze an unprecedented amount of data will be the key to success for every industry.
To exploit the benefits of a big data strategy, a key question is how to translate all of that data into useful knowledge. To meet this challenge, a company first needs to have a clear picture of their strategic knowledge assets, such as their area of expertise, core competencies, and intellectual property.
Having a clear picture of the business model and the relationships with distributors, suppliers, and customers is extremely useful in order to design a tactical and strategic decision-making process. The true potential value of big data is only gained when placed in a business context, where data analysis drives better decisions — otherwise, it’s just data.
In a new O’Reilly report Data Preparation in the Big Data Era, we provide a step-by-step guide to manage the challenges of data cleaning and preparation — critical steps before effective data analysis can begin. We explore the common problems of data preparation and the different steps involved, including data cleaning, combination, and transformation. You’ll also learn about new products that deal with problem of data variety at scale, including Tamr’s solution, which curates data at scale using a combination of machine learning and expert feedback. Read more…
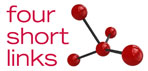

Four short links: 25 September 2015
Predicting Policing, Assaulting Advertising, Compliance Ratings, and $9 Computer
- Police Program Aims to Pinpoint Those Most Likely to Commit Crimes (NYT) — John S. Hollywood, a senior operations researcher at the RAND Corporation, said that in the limited number of studies undertaken to measure the efficacy of predictive policing, the improvement in forecasting crimes had been only 5% or 10% better than regular policing methods.
- Apple’s Assault on Advertising and Google (Calacanis) — Google wants to be proud of their legacy, and tricking people into clicking ads and selling our profiles to advertisers is an awesome business – but a horrible legacy for Larry and Sergey. Read beside the Bloomberg piece on click fraud and the future isn’t too rosy for advertising. If the ad bubble bursts, how much of the Web will it take with it?
- China Is Building The Mother Of All Reputation Systems To Monitor Citizen Behavior — The document talks about the “construction of credibility” — the ability to give and take away credits — across more than 30 areas of life, from energy saving to advertising.
- $9 Computer Hardware (Makezine) — open hardware project, with open source software. The board’s spec is a 1GHz R8 ARM processor with 512MB of RAM, 4GB of NAND storage, and Wi-Fi and Bluetooth built in.

Training in the big data ecosystem
The O'Reilly Radar Podcast: Paco Nathan and Jesse Anderson on the evolution of the data training landscape.
Subscribe to the O’Reilly Radar Podcast to track the technologies and people that will shape our world in the years to come.
In this week’s Radar Podcast, O’Reilly’s Ben Lorica talks to Paco Nathan, director of O’Reilly Learning, and Jesse Anderson, technical trainer and creative engineer at Confluent.
Their discussion focuses on the training landscape in the big data ecosystem, their teaching techniques and particular content they choose, and a look at some expected future trends.
Here are a few snippets from their chat:
Training vs PowerPoint slides
Anderson: “Often, when you have a startup and somebody says, ‘Well, we need some training,’ what will usually happen is one of the software developers will say, ‘OK, I’ve done some training in the past and I’ll put together some PowerPoints.’ The differences between a training thing and doing some PowerPoints, like at a meetup, is that a training actually has to have hands-on exercises. It has to have artifacts that you use right there in class. You actually need to think through, these are concepts, these are things that the person will need to be successful in that project. It really takes a lot of time and it takes some serious expertise and some experience in how to do that.”
Nathan: “Early on, you would get some committer to go out and do a meetup, maybe talk about an extension to an API or whatever they were working on directly. If there was a client firm that came up and needed training, then they’d peel off somebody. As it evolved, that really didn’t work. That kind of model doesn’t scale. The other thing too is, you really do need people who understand instructional design, who really understand how to manage a classroom. Especially when it gets to any size, it’s not just a afterthought for an engineer to handle.” Read more…

Building enterprise data applications with open source components
The O’Reilly Data Show podcast: Dean Wampler on bounded and unbounded data processing and analytics.
Subscribe to the O’Reilly Data Show Podcast to explore the opportunities and techniques driving big data and data science.
I first found myself having to learn Scala when I started using Spark (version 0.5). Prior to Spark, I’d peruse books on Scala but just never found an excuse to delve into it. In the early days of Spark, Scala was a necessity — I quickly came to appreciate it and have continued to use it enthusiastically.
For this Data Show Podcast, I spoke with O’Reilly author and Typesafe’s resident big data architect Dean Wampler about Scala and other programming languages, the big data ecosystem, and his recent interest in real-time applications. Dean has years of experience helping companies with large software projects, and over the last several years, he’s focused primarily on helping enterprises design and build big data applications.
Here are a few snippets from our conversation:
Apache Mesos & the big data ecosystem
It’s a very nice capability [of Spark] that you can actually run it on a laptop when you’re developing or working with smaller data sets. … But, of course, the real interesting part is to run on a cluster. You need some cluster infrastructure and, fortunately, it works very nicely with YARN. It works very nicely on the Hadoop ecosystem. … The nice thing about Mesos over YARN is that it’s a much more flexible, capable resource manager. It basically treats your cluster as one giant machine of resources and gives you that illusion, ignoring things like network latencies and stuff. You’re just working with a giant machine and it allocates resources to your jobs, multiple users, all that stuff, but because of its greater flexibility, it cannot only run things like Spark jobs, it can run services like HDFS or Cassandra or Kafka or any of these tools. … What I saw was there was a situation here where we had maybe a successor to YARN. It’s obviously not as mature an ecosystem as the Hadoop ecosystem but not everybody needs that maturity. Some people would rather have the flexibility of Mesos or of solving more focused problems.

Major players and important partnerships in the big data market
A fully data-driven market report maps and analyzes the intersections between companies.
Download our new free report “Mapping Big Data: A Data Driven Market Report” for insights into the shape and structure of the big data market.
Who are the major players in the big data market? What are the sectors that make up the market and how do they relate? Which among the thousands of partnerships are most important?
These are just a handful of questions we explore in-depth in the new O’Reilly report now available for free download: Mapping Big Data: A Data Driven Market Report. For this new report, San Francisco-based startup Relato mapped the intersection of companies throughout the data ecosystem — curating a network with tens of thousands of nodes and edges representing companies and partnerships in the big data space.
Relato created the network by extracting data from company home pages on the Web and analyzed it using social network analysis; market experts interpreted the results to yield the insights presented in the report. The result is a preview of the future of market reports. Read more…