- Eve, Version 0 (Chris Grainger) — Version 0 contains a database, compiler, query runtime, data editor, and query editor. Basically, it’s a database with an IDE. You can add data both manually or through importing a CSV and then you can create queries over that data using our visual query editor.
- BOOM: Berkeley Orders Of Magnitude — an effort to explore implementing Cloud software using disorderly, data-centric languages.
- Eigenstyle — clever analysis and reconstruction of images through principal component analysis. And here are “prettiest ugly dresses,” those that I classified as dislikes, that the program predicted I would really like.
- Turing Digital Archive — many of Turing’s letters, talks, photographs, and unpublished papers, as well as memoirs and obituaries written about him. It contains images of the original documents that are held in the Turing collection at King’s College, Cambridge. (Timely as Jason Scott works to save a manual archive: [1], [2], [3])
"Big Data" entries

Unsupervised learning, attention, and other mysteries
How to almost necessarily succeed: An interview with Google research scientist Ilya Sutskever.
Get notified when our free report “Future of Machine Intelligence: Perspectives from Leading Practitioners” is available for download. The following interview is one of many that will be included in the report.
Ilya Sutskever is a research scientist at Google and the author of numerous publications on neural networks and related topics. Sutskever is a co-founder of DNNresearch and was named Canada’s first Google Fellow.
Key Takeaways:
- Since humans can solve perception problems very quickly, despite our neurons being relatively slow, moderately deep and large neural networks have enabled machines to succeed in a similar fashion.
- Unsupervised learning is still a mystery, but a full understanding of that domain has the potential to fundamentally transform the field of machine learning.
- Attention models represent a promising direction for powerful learning algorithms that require ever less data to be successful on harder problems.
David Beyer: Let’s start with your background. What was the evolution of your interest in machine learning, and how did you zero-in on your Ph.D. work?
Ilya Sutskever: I started my Ph.D. just before deep learning became a thing. I was working on a number of different projects, mostly centered around neural networks. My understanding of the field crystallized when collaborating with James Martens on the Hessian-free optimizer. At the time, greedy layer-wise training (training one layer at a time) was extremely popular. Working on the Hessian-free optimizer helped me understand that if you just train a very large and deep neural network on a lot of data, you will almost necessarily succeed. Read more…

A “bottom-up” approach to data unification
How machine learning plus expert sourcing can unify customer data at scale.
Watch the free webcast Integrating Customer Data at Scale to learn how Toyota Motor Europe was able to unify its customer data at scale.
Enterprises that are capable of gaining a unified view of their customer data can achieve added business enhancements and user opportunities. Capturing customer data, however, can be a difficult task, as most systems rely on traditional “top-down” approaches to standardizing data. In a recent O’Reilly webcast, Integrating Customer Data at Scale, Tamr field engineer Alan Wagner hosts a Q&A session with Matt Stevens, the general manager at Toyota Motor Europe, to demonstrate how a leading enterprise uses a third-generation system like Tamr to simplify the process of unifying customer data.
In the webcast, Stevens explains how Toyota Motor Europe has gained a 360-degree view of their customers through the Tamr Data Unification Platform, which takes a machine learning and expert-sourcing “human guided workflow” approach to data unification. Wagner provides a demo of the Tamr platform, applied within a Salesforce application, to demonstrate the ability to capture and unify customer data. Read more…
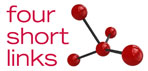

Four short links: 18 August 2015
Chris Grainger Ships, Disorderly Data-Centric Languages, PCA for Fun and Fashion, and Know Thy History

Pattern recognition and sports data
The O'Reilly Data Show Podcast: Award-winning journalist David Epstein on the (data) science of sports.
Sign-up now to receive a free download of the new O’Reilly report “Data Analytics in Sports: How Playing with Data Transforms the Game” when it publishes this fall.
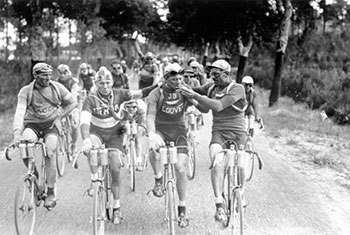
Julien Vervaecke and Maurice Geldhof smoking a cigarette at the 1927 Tour de France. Public domain photo via Wikimedia Commons.
In a recent episode of the O’Reilly Data Show Podcast, I spoke with Epstein about his book, data science and sports, and his recent series of articles detailing suspicious practices at one of the world’s premier track and field training programs (the Oregon Project).
Nature/nurture and hardware/software
Epstein’s book contains examples of sports where athletes with certain physical attributes start off with an advantage. In relation to that, we discussed feature selection and feature engineering — the relative importance of factors like training methods, technique, genes, equipment, and diet — topics which Epstein has written about and studied extensively:
One of the most important findings in sports genetics is that your ability to improve with respect to a certain training program is mediated by your genes, so it’s really important to find the kind of training program that’s best tailored to your physiology. … The skills it takes for team sports, these perceptual skills, nobody is born with those. Those are completely software, to use the computer analogy. But it turns out that once the software is downloaded, it’s like a computer. While your hardware doesn’t do anything alone without software, once you’ve got the software, the hardware actually makes a lot of a difference in how good of an operating machine you have. It can be obscured when people don’t study it correctly, which is why I took on some of the 10,000 hours stuff. Read more…

We make the software, you make the robots
An interview with Andreas Mueller, on scikit-learn and usable machine learning software.
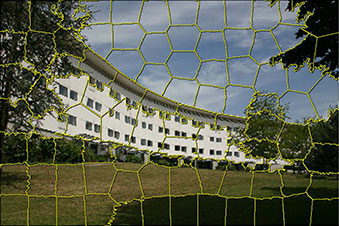
Superpixels example from Andreas Mueller’s thesis paper (PDF), used with permission.
Mueller wears many hats at work. He is one of the key maintainers of the popular Python machine learning library scikit-learn. Holding a doctorate in computer vision from the University of Bonn in Germany, he currently works on open science at New York University’s Center for Data Science. He speaks at conferences around the world and has a fanbase of 5,000+ followers on Twitter and about as many reputation points on Stack Overflow. In other words, this man has got mad street cred. He started out doing pure math in academia, and has now achieved software developer cult idol status. Read more…
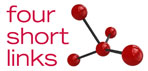

Four short links: 11 August 2015
Real-time Sports Analytics, UI Regression Testing, AI vs. Charity, and Google's Data Pipeline Model
- Denver Broncos Testing In-Game Analytics — their newly hired director of analytics working with the coach. With Tanney nearby, Kubiak can receive a quick report on the statistical probabilities of almost any situation. Say that you have fourth-and-3 from the opponent’s 45-yard-line with four minutes to go. Do the large-sample-size percentages make the risk-reward ratio acceptable enough to go for it? Tanney’s analytics can provide insight to aid Kubiak’s decision-making. (via Flowing Data)
- Visual Review (GitHub) — Apache-licensed productive and human-friendly workflow for testing and reviewing your Web application’s layout for any regressions.
- Effective Altruism / Global AI (Vox) — fear of AI-run-amok (“existential risks”) contaminating a charity movement.
- The Dataflow Model (PDF) — Google Research paper presenting a model aimed at ease of use in building practical, massive-scale data processing pipelines.

The world beyond batch: Streaming 101
A high-level tour of modern data-processing concepts.
Editor’s note: This is the first post in a two-part series about the evolution of data processing, with a focus on streaming systems, unbounded data sets, and the future of big data. See part two.
Streaming data processing is a big deal in big data these days, and for good reasons. Amongst them:
- Businesses crave ever more timely data, and switching to streaming is a good way to achieve lower latency.
- The massive, unbounded data sets that are increasingly common in modern business are more easily tamed using a system designed for such never-ending volumes of data.
- Processing data as they arrive spreads workloads out more evenly over time, yielding more consistent and predictable consumption of resources.
Despite this business-driven surge of interest in streaming, the majority of streaming systems in existence remain relatively immature compared to their batch brethren, which has resulted in a lot of exciting, active development in the space recently.
As someone who’s worked on massive-scale streaming systems at Google for the last five+ years (MillWheel, Cloud Dataflow), I’m delighted by this streaming zeitgeist, to say the least. I’m also interested in making sure that folks understand everything that streaming systems are capable of and how they are best put to use, particularly given the semantic gap that remains between most existing batch and streaming systems. To that end, the fine folks at O’Reilly have invited me to contribute a written rendition of my Say Goodbye to Batch talk from Strata + Hadoop World London 2015. Since I have quite a bit to cover, I’ll be splitting this across two separate posts:
- Streaming 101: This first post will cover some basic background information and clarify some terminology before diving into details about time domains and a high-level overview of common approaches to data processing, both batch and streaming.
- The Dataflow Model: The second post will consist primarily of a whirlwind tour of the unified batch + streaming model used by Cloud Dataflow, facilitated by a concrete example applied across a diverse set of use cases. After that, I’ll conclude with a brief semantic comparison of existing batch and streaming systems.
So, long-winded introductions out of the way, let’s get nerdy. Read more…

What it means to “go pro” in data science
A look at what it takes to be a professional data science programmer.
My experience of being a data scientist is not at all like what I’ve read in books and blogs. I’ve read about data scientists working for digital superstar companies. They sound like heroes writing automated (near sentient) algorithms constantly churning out insights. I’ve read about MacGyver-like data scientist hackers who save the day by cobbling together data products from whatever raw material they have around.
The data products my team creates are not important enough to justify huge enterprise-wide infrastructures. It’s just not worth it to invest in hyper-efficient automation and production control. On the other hand, our data products influence important decisions in the enterprise, and it’s important that our efforts scale. We can’t afford to do things manually all the time, and we need efficient ways of sharing results with tens of thousands of people.
There are a lot of us out there — the “regular” data scientists; we’re more organized than hackers but with no need for a superhero-style data science lair. A group of us met and held a speed ideation event, where we brainstormed on the best practices we need to write solid code. This article is a summary of the conversation and an attempt to collect our knowledge, distill it, and present it in one place. Read more…

Hadoop for business: Analytics across industries
The O’Reilly Podcast: Ben Sharma on the business impact of Hadoop and the evolution of tools
In this episode of the O’Reilly Podcast, O’Reilly’s Ben Lorica chats with Ben Sharma, CEO and co-founder of Zaloni, a company that provides enterprise data management solutions for Hadoop. Sharma was one of the first users of Apache Hadoop, and has a background in enterprise solutions architecture and data analytics.
Before starting Zaloni, Sharma spent many years as a business consultant and began to see that companies across industries were struggling to process, store, and extract value from their data. Having worked extensively in telecom, Sharma helped equipment vendors deploy large-scale network infrastructures at carriers across the world. He began to see how Hadoop could have an impact in the business analytics aspect of companies, not just in IT.
In this interview, Lorica and Sharma discuss the early days of Hadoop and how businesses across industries are benefitting from Hadoop. They also discuss the evolution of tools in the space and how more companies are moving toward real-time decision-making with the growth of streaming tools and real-time data. Read more…

How an enterprise begins its big data journey
An ETL offload solution addresses the challenges of data overload, rising costs, and the skills gap.
As the amount of data continues to double in size every two years, organizations are struggling more than ever before to manage, ingest, store, process, transform, and analyze massive data sets. It has become clear that getting started on the road to using data successfully can be a difficult task, especially with a growing number of new data sources, demands for fresher data, and the need for increased processing capacity. In order to advance operational efficiencies and drive business growth, however, organizations must address and overcome these challenges.
In recent years, many organizations have heavily invested in the development of enterprise data warehouses (EDW) to serve as the central data system for reporting, extract/transform/load (ETL) processes, and ways to take in data (data ingestion) from diverse databases and other sources both inside and outside the enterprise. Yet, as the volume, velocity, and variety of data continues to increase, already expensive and cumbersome EDWs are becoming overloaded with data. Furthermore, traditional ETL tools are unable to handle all the data being generated, creating bottlenecks in the EDW that result in major processing burdens.
As a result of this overload, organizations are now turning to open source tools like Hadoop as cost-effective solutions to offloading data warehouse processing functions from the EDW. While Hadoop can help organizations lower costs and increase efficiency by being used as a complement to data warehouse activities, most businesses still lack the skill sets required to deploy Hadoop. Read more…